
The progress of this last year regarding Deep Learning is truly exceptional. Many steps forward have been made in many fields of technology thanks to neural networks and among these there is the synthetic voice, or rather the Text-To-Speech (TTS) that is, that series of technologies able to simulate the human way of speaking by reading a text. Among the models realized, therefore, there is WaveNet, a highly innovative model that has revolutionized the way of doing Text-To-Speech making them jump really forward.
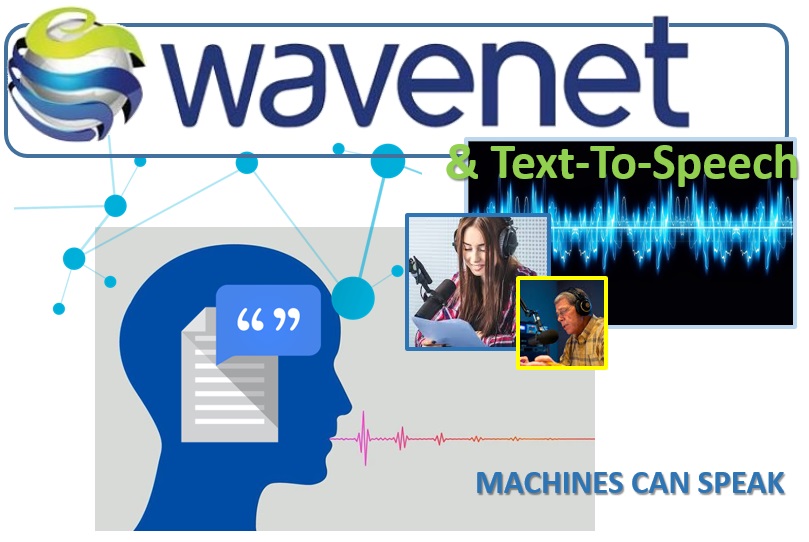
Text-To-Speech technologies (TTS)
The desire to make the machines speak simulating human beings as much as possible has always been a dream for all researchers. In recent years, the numerous applications of “talking machines” in many professional fields have strongly pushed the research and the market to look for increasingly efficient solutions that would produce increasingly real results.
But the realization of a talking machine has not proved an easy thing, the human way of speaking is very complex, and it is not only made of recorded words, but also inflections, expressions that add emphasis and emotions to the pronunciation, so as to make it understandable or not. An aseptic word in a sentence without expressions can make the result not only unpleasant but also difficult to understand.
However, many results have been seen, so as to develop a new technological area called Text-to-Speech (TTS), or less commonly Speech Synthesis.
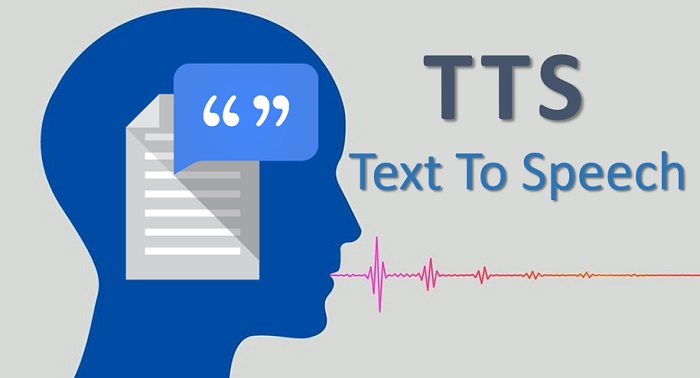
Most devices that need this technology base their functioning on the concatenative TTS. This technique uses a large database of small fragments of speech (recorded words) from a single speaker and then recombines with each other to form whole sentences. Satellite navigators, mobile phones, automated voices in train stations use this technique. However, despite its considerable diffusion, the concatenative TTS is very limited. In fact there are no inflections of the voice as emphasis and emotions, and are based totally on a single voice. If you wanted a different voice it would be necessary to re-record all the words in a new database.
These limitations have led to the development of the parametric TTS technique, where all the information necessary to generate the data is stored in the parameters of a model, and therefore the contents and characteristics of the speech mode can be controlled by input passed directly to the model. The model is then based on the parameters to generate and process the audio signals, using numerous algorithms for signal processing (signal processing) called vocoders.
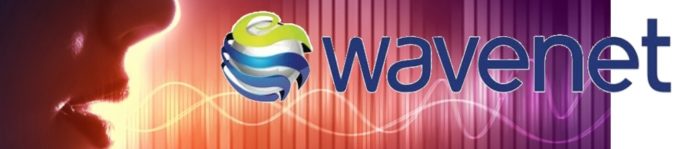
WaveNet
Completely different from the two previous TTS technologies, WaveNet works directly modeling the waveform of the audio signal, one sample at a time. This technology is therefore not limited to Text-To-Speech applications but can also be used to generate any type of audio, including music.
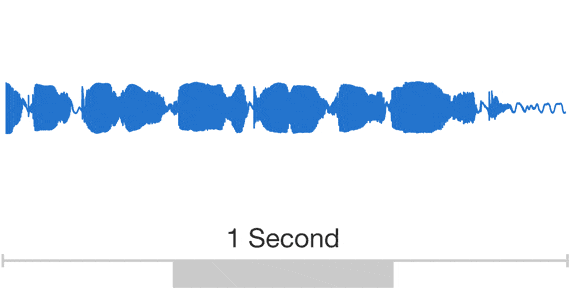
Researchers have always tried to avoid creating audio waveform patterns for a valid reason: they are extremely fast. In fact, for every second of sound wave several thousand samples are needed, and with regard to the modeling structures can be highlighted for any time scale, making it almost impossible to simplify the model.
However, with the progress made by Deep Learning in recent years, it has been possible to realize a model capable of working directly at the level of a single sample. WaveNet is based on the construction of a completely self-regressive model, in which the prediction of a single sample is the result of the previous ones already worked out.
This was possible thanks to the successes of two Deep Learning models, called PixelRNN e PixelCNN, made just this last year. Created for the generation of natural images, they are based on the construction of every single pixel (and its colors) based on the calculated prediction taking into account all the other surrounding pixels. The success of these two models, which work on two-dimensional systems, has inspired researchers to use WaveNet, which is the one-dimensional adaptation of the two previous models, applying it in the field of audio waves.

The animation above shows how WaveNet works to generate predictions for each individual sample. The WaveNet structure is based on a completely convolutional neural, where convolution layers have various factors of delation that allow its respective fields to grow exponentially with depth and cover thousands of timesteps.
During the learning period of the neural network (training time), real sound waves samples recorded by human speakers are used as input sequences.
WaveNet is the current most advanced system for TTS
Once the model was realized by the researchers, tests were immediately carried out to evaluate their performance.
First of all WaveNet was trained using Google’s TTS datasets in order to evaluate its performance, remaining in the same field of the other two TTS techniques currently used by Google (parametric and concatenative).
Then it was decided to compare all the methods, including a real human speech, through the Mean Opinion Scores (MOS). MOSs are a standard measure for testing sound quality, and are conducted as blind tests with human subjects, who have the ability to grade from 1 to 5.
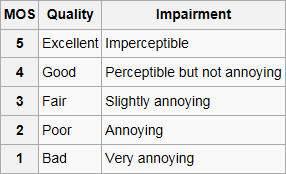
As for the languages, both US English and Chinese Mandarin were used, a very complex language. However, the results were similar in both cases.
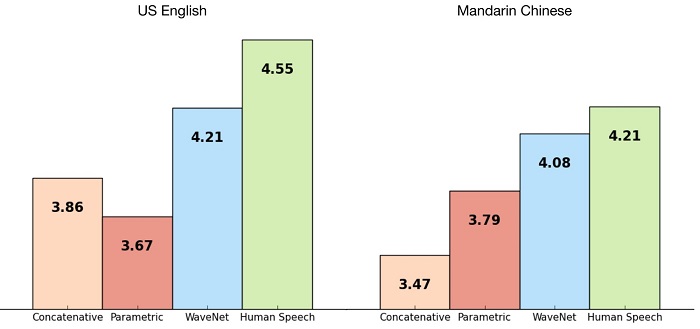
From the results obtained, it can also be seen that with WaveNet the perception of synthesis and natural of the human voice has been greatly reduced by over 50%. This is perhaps the most outstanding result of this technology.
Let’s test WaveNet
Here are some examples of these three systems that you can listen to and evaluate yourself.
US English:
Mandarin Chinese:
Adding reading texts for WaveNet
Once the model for speech synthesis had been realized, the next step was to use it using text as input. So we had to find a way to teach WaveNet what a text was.
To do this, the best practice was to convert a written text into a sequence of phonetic and linguistic characteristics (which contain information on phoneme, syllable, word, etc.) and using them as input to be associated with the corresponding sound waves.
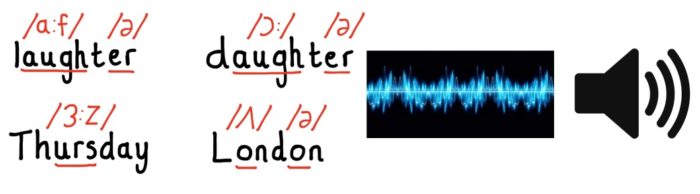
So WaveNet has also developed so that the neural network is able to make predictions conditioned not only by previous audio samples, but also by the text passed in reading.
An interesting experiment is to have WaveNet generate a speech without the introduction of an attached text. The result was surprising, given that there is still the generation of a spoken language, but that listening is a stutter where words are barely mentioned, truncated creating discourses without meaning.
Also listening to the previous results, you can also hear sounds that are not directly related to the pronunciation of words, but come from the breath and the moment of the mouth. This is certainly a good indication of the great flexibility of the WaveNet model to generate realistic audio.
Using multiple speakers in an easy way
Another step forward is that WaveNet is able to learn features from many different voices, both male and female. In order to avoid problems with the reproduction of a specific synthetic voice, WaveNet is conditioned to identify the speaker that is speaking, so that it can be played correctly.
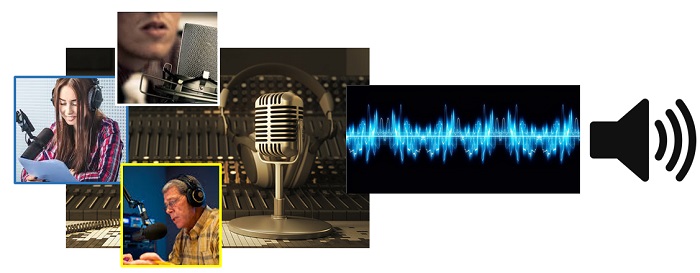
With this approach, it has been shown that learning from each speaker has proved easier when the neural network learns from several different speakers, compared to when learning is based on a single speaker. This phenomenon highlights a factor of trans-learning between the various speakers. That is what WaveNet learns from a speaker can be used for others.
This aspect is really stunning.
Eventually you can change the speaker’s identity to tell WaveNet which voice to use for playing the same text.
WaveNet is not limited to just TTS, but can also generate music
Based on the prediction of individual audio samples, we have also seen that WaveNet is able to model any other audio signal, in addition to human voices. We therefore tried to use it in other areas. Interesting for example are the attempts made using melodies of classical music played only with a piano.
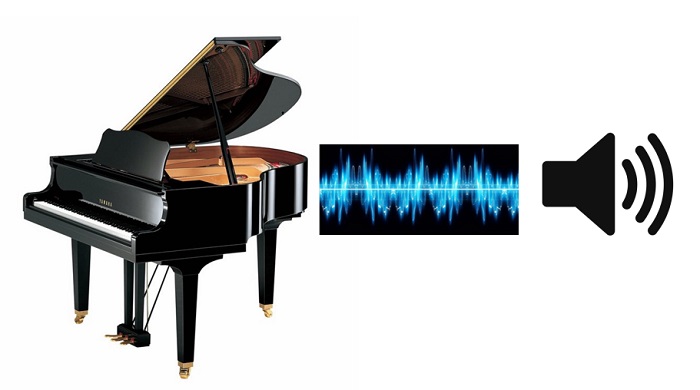
Really fascinating results have been obtained.
Conclusions
2017 proved to be the year of Deep Learning, where many prediction techniques and models were presented and demonstrated their great predictive skills. Also the Text-To-Speech has seen the introduction of WaveNet, a system able to simulate the human way of speaking in a stunning way, bringing human perception to almost no longer distinguish between a synthetic and a natural voice.
We hope that 2018 can bring many innovations in this and many other fields.
[:]